Researchers use GPTZero to investigate the impact of LLMs in peer reviews
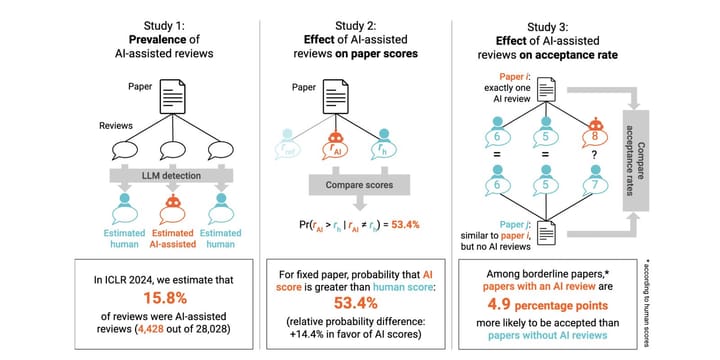
In the ever-evolving landscape of academia, the integrity of the peer-review process stands as a pillar of scientific advancement. It’s used by journals and conferences to ensure the validity and significance of research findings and funding institutions to allocate research grants. Peer-reviewed research is prioritized by policy advisory groups, holds special status in the courtroom, and is required for researchers to progress in their academic careers.
However, the rapidly growing number of researchers and papers published is outpacing the number of qualified reviewers. “Between 2022 to 2023, the number of submissions increased by 26%. Meanwhile, the number of reviewers increased by 20%.”
In a recent study, researchers used GPTZero to investigate the growing concern of the impact of LLMs on the scientific process. Led by Giuseppe Russo, Manoel Horta Ribeiro, Tim R. Davidson, Veniamin Veselovsky and Robert West, the study analyzes the consequences of AIAI-assisted peer reviews.
Key findings
- At least 15.8% of peer reviews were written using AI assistance
- AI-assisted reviews had a 14.4% increased odds of assigning higher scores than human reviews
- Borderline papers receiving an AI-assisted peer review were 4.9% more likely to be accepted than papers that did not
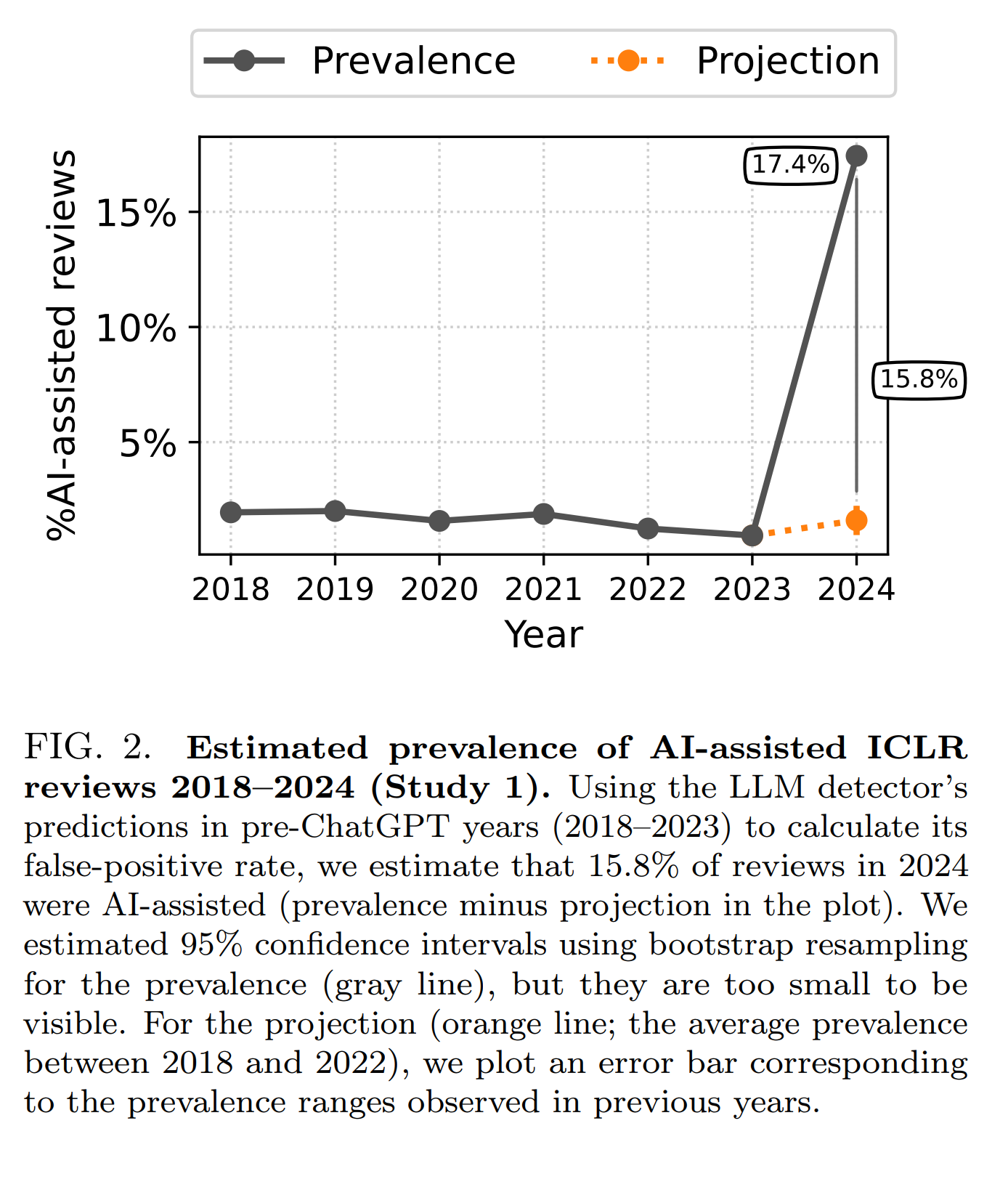
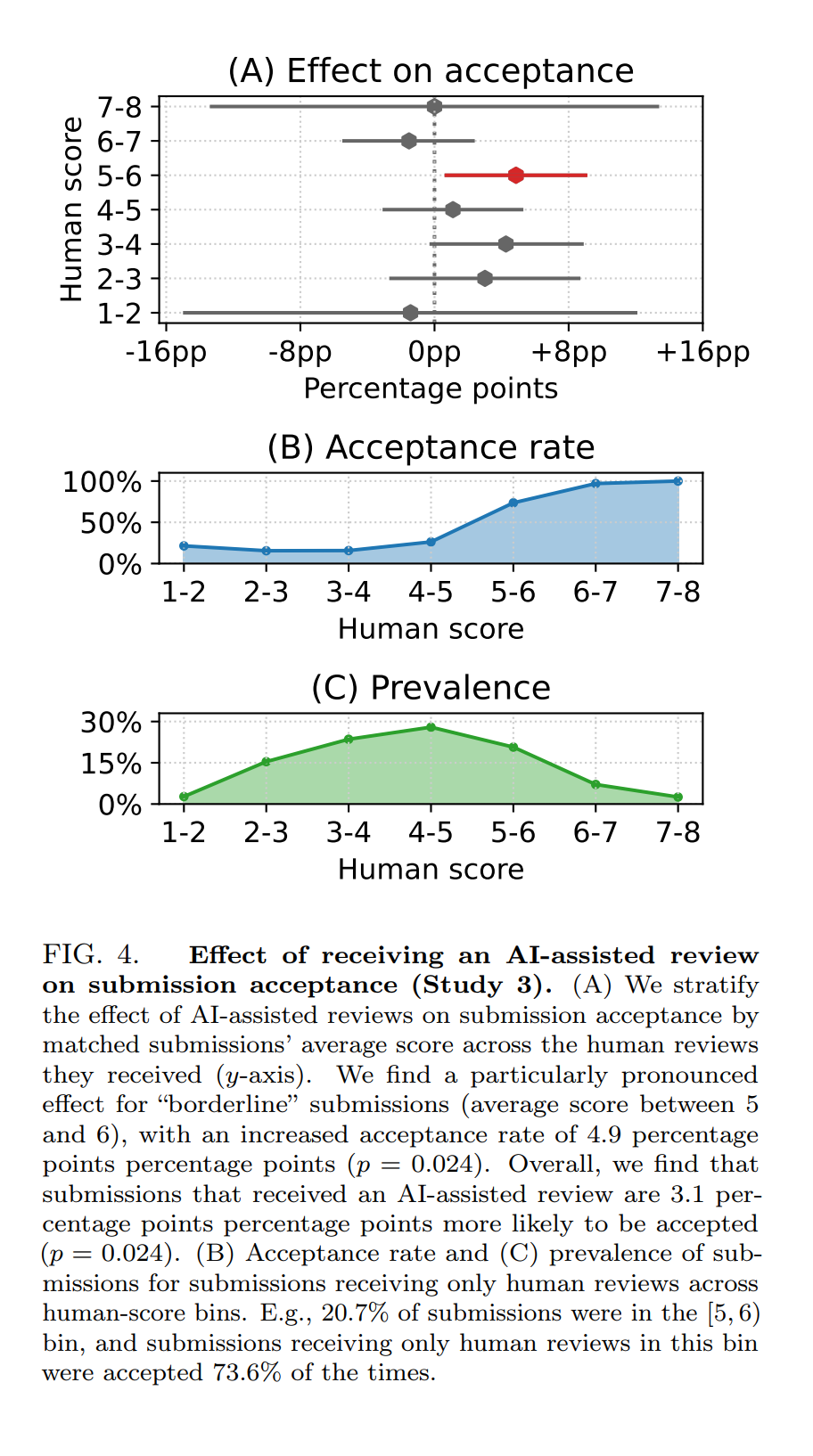
What do these findings mean? Peer reviews assisted by LLMs may impact the validity and fairness of the peer review system, one of the cornerstones of modern science.
Understanding the landscape
The research focuses on reviews from the International Conference on Learning Representations (ICLR) between 2018 and 2024. With the help of GPTZero, an AI detection tool, the team estimated the prevalence of AI-assisted reviews, revealing that at least 15.8% of reviews were written using a LLM.
The impact of using AI in peer reviews
Employing a quasi-experimental approach, the researchers studied the consequences of AI-assisted peer reviews. They found that on average, AI-assisted reviews had 14.4% increased odds of boosting scores and increased the likelihood of borderline paper acceptance by 4.9%. In particular the effect on borderline papers tangible impact on the fate of submissions.
Implications
“Reduced reviewer input could lead to papers’ scientific merit being judged incorrectly. Scientists’ reliance on LLMs to write peer reviews could thus decrease the peer reviewers system’s quality and harm its social and epistemic functions. In response, multiple journals and conferences felt obliged to regulate or outright prohibit the use of LLMs in the scientific process,” the study mentioned. “It is important to note that using LLMs in reviewing isn’t all bad. In fact, as the authors note, there are many areas where an LLM could actually improve the current reviewing ecosystem! In general, improvements can be seen in both the existing reviewing pipeline and offering previously unseen tools to potentially improve peer review. For the former, they “could offer reviewers feedback to improve writing clarity, detect flawed critiques to reduce misunderstanding or help contextualize the importance of a submission’s findings”. For the latter, “they might even provide new ways to tackle problems poorly addressed by the current peer-review process, e.g., by conducting automated tests to alleviate the reproducibility crisis.”
Moving forward, the researchers suggested rethinking the reporting requirements of the integration of AI in peer reviews to uphold the integrity of the scientific process.
Interested in getting research access to our API and collaborating with the GPTZero team? Please reach out to us!