ESL Bias in AI Detection is an Outdated Narrative
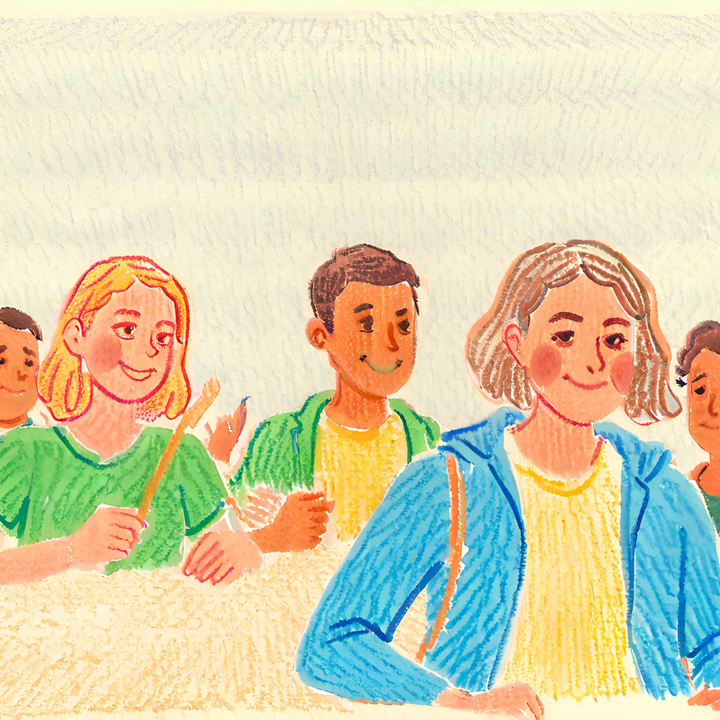
Recently, we've seen the resurfacing of a previous narrative on (social) media that AI identification is biased against and failing ESL students. But this is an outmoded view from out-of-date research.
In this article, we revisit the original study on ESL bias from Stanford University, and discuss the progress GPTZero has made in mitigating detection bias. Finally we replicate and re-run the original study with the same dataset and parameters outlined by Stanford researchers to demonstrate conclusively that GPTZero’s current and improved detection methods does not exhibit classification bias against ESL written text.
Transparent Evaluations
First and foremost, we are strongly supportive of the work of independent and academic reviewers in evaluating the progress of AI models. Starting in March, the GPTZero team launched our AI transparency efforts by providing API access to our model free-of-charge to academic researchers, including researchers from MIT, Harvard, Stanford, and several other universities.
The GPTZero Machine Learning team has also made several original contributions to the field, including introducing burstiness and writing variation as an effective AI discriminator, introducing perplexity based classification in January, and developing the first sentence-specific AI highlighting model in February.
The Stanford ESL study was conducted six months ago in April, arguably a lifetime in artificial intelligence time. The researchers evaluated seven AI classification models on ninety-one texts from the TOEFL (test of english as a foreign language proficiency) examination and on eighty-eight US middle school essays. GPTZero performed the best of any classifier — perfect — in classifying middle school essays. All seven of the classifiers performed poorly in classifying the TOEFL essay dataset.
However, on October 19th, we re-ran the author’s own code on our current AI models. Our current AI identification model classified 1 of the 91 ESL texts as AI-generated. These results conclusively passed the study's parameters for bias against ESL detection, and was an astronomical improvement from April.
The current GPTZero model classified 1.1% of the results as AI generated, and flagged 6.6% as uncertain or possible AI. The dataset and open-sourced Github repository of the original Stanford ESL study is linked here, as is our GPTZero evaluation data, for reproducibility purposes.
De-biasing Research and Progress
The notable improvements above are the result of our ML team's dedicated research on de-biasing AI classification models — efforts that started in April, and included regular model updates and improvements, which have translated into a noticeable impact six months later.
Our efforts in reducing ESL bias in classification can be categorized as either a) model parameter tagging, b) text pre-classification, or c) representative dataset insertions.
In parameter tagging, we experimented with an additional CNN-layer that incorporated tagged information during model training — including an 'education tag.' This method enables an AI identification model to focus its results on an education-and-ESL related corpus of texts rather than only considering the larger corpus of journalism, news, and business writing and their patterns. Text pre-classification is a similar concept but during the model output step rather than the during model training step. Through training a classification model, we can predict beforehand whether a text is likely from an ESL writer, to ensure the AI identification model has this information when making a classification.
Representative Datasets
The most critical aspect to improving our models for ESL data was making sure that ESL writers were represented in the model training data.
To this goal, we added global news, scientific articles, and various datasets from international writers that provide diverse ESL contexts. Our collection includes specified TOEFL examination datasets, a 180k Medium articles dataset, a 31k Persuade articles set, and a 12k article dataset from the Hewlett Foundation. Instead of solely focusing on full documents, we now also train on individual sentences, boosting performance on shorter texts. This method is comparable to random data augmentation, sampling random sentences from each document.
Conclusions: Better than Better
With GPTZero's commitment to being the 'gold standard' for AI identification we are rigorously committed to continuing our research in de-biasing AI detection.
We understand there is a limitation to our team's abilities in delivering model improvements in short time periods. As such, we are are also committed to lateral improvements beyond research metrics, including better model interpretability, better evaluations, and better guidelines for AI adoption.
In terms of evaluations, several of our ML researchers noted that the evaluation dataset provided by the Stanford researchers was not rigorous enough due to dataset size. (91 and 88 examples are a fraction of 1+ million articles in the GPTZero model training set). As such the GPTZero Machine Learning team has collected a more rigorous evaluation dataset consisting of TOEFL, CS22N, Hewlett Foundation, and US College Application Essays. In this large scale ESL dataset, the improved GPTZero model also performed with a <2% false positive rate.
Figure 1: Output probability distribution showing performance metrics on ESL data for the September GPTZero model compared to the October GPTZero model. It is clear that the current model is significantly better than the previous one. The October model has far fewer false positives indicated by the separation of the AI and Human predications.
In terms of adoption, the GPTZero team is especially conscious of the special relationship between educators and students, and have committed to introducing interpretability metrics and resources for understanding AI identification tools. More so, we are partnering with the American Federation of Teachers and other educational organizations to introduce guidelines for positive use and adoption of AI identification in education.