GPTZero Public Letter to White House Presidential Advisory Committee
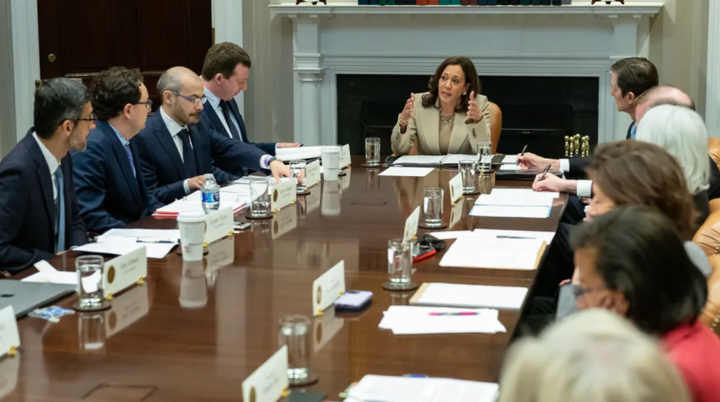
On July 21, the White House announced that OpenAI, Google, and other actors pledged to watermark AI content for safety after notable high profile policy discussions. In response, GPTZero and affiliate researchers have submitted our own recommendations to the White House on responsible AI adoption and detection.
Our full recommendations are released and now available to the public below.
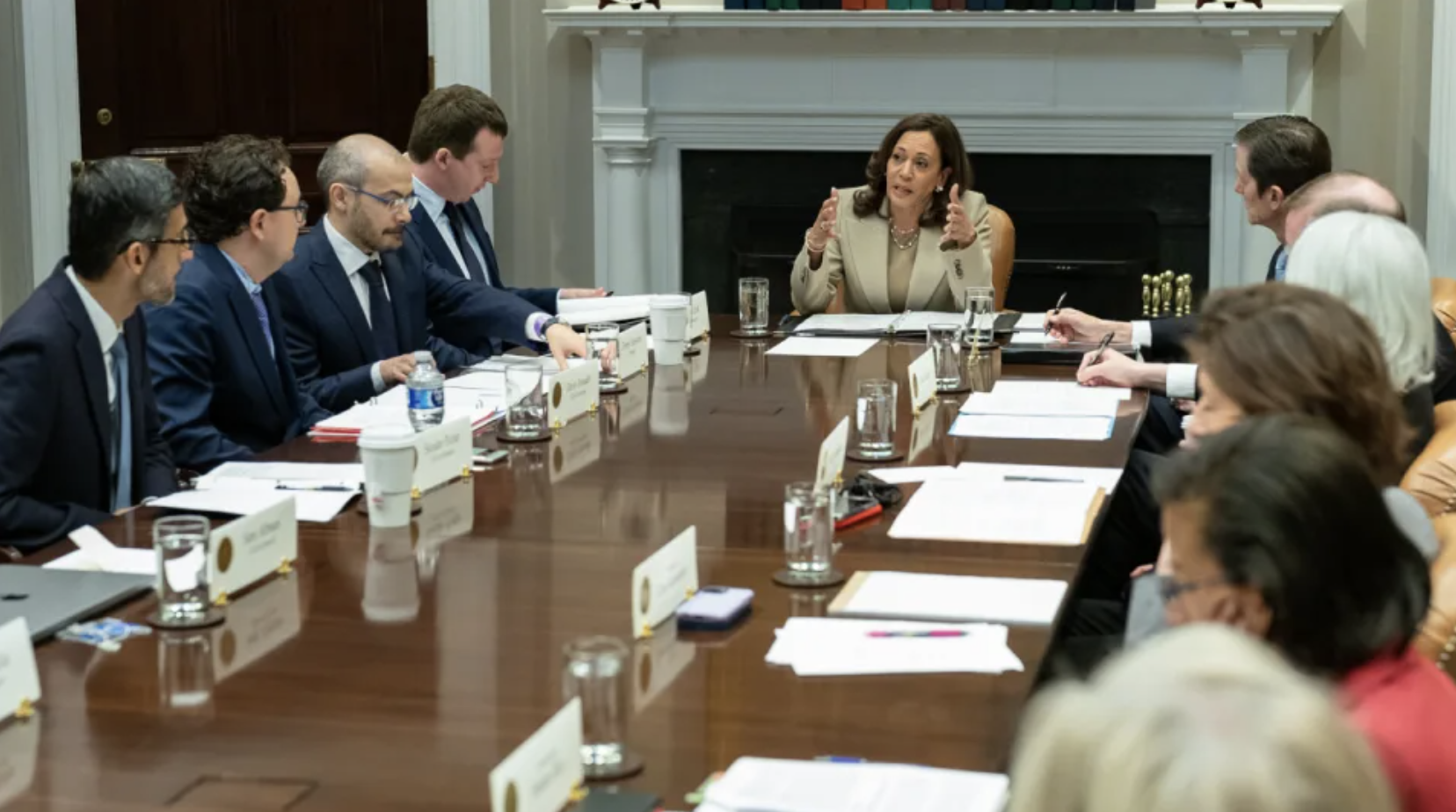
President’s Council of Advisors on Science and Technology
July 23, 2023
Thank you for the opportunity to submit input to the Working Group on Generative AI. We are a team of researchers from GPTZero, Princeton University’s Center for Information Technology Policy, and the University of Oxford writing to offer suggestions on the role of AI-generated text detection systems in addressing the problems of malicious actors manipulating people’s beliefs and AI-generated noise drowning out valuable public discourse.
AI can cheaply produce content that is convincing (uses sophisticated, error-free language), impersonating (copies the style of trusted sources and individuals), interactive (responds to users promptly), and targeted (tailors content to a particular user). These capabilities make it harder for people to discern what to read and trust online, as common indicators of trustworthy information become less reliable. We believe that defending against these harms will likely require embracing a principle reflected in California’s chatbot disclosure mandates: users ought to know when they are interacting with an AI system or AI-generated content.
In this comment, we provide:
- An overview of relevant channels through which harms can propagate;
- A review of the state-of-the-art techniques for detecting AI-generated text;
- A description of the costs of implementing AI-text detection systems;
- A menu of policy and technical interventions for PCAST to consider.
AI-generated text may be used to manipulate people or drown out discourse through five main channels.
- Organizations that rely on public input: Congress members, special committees, and this very working group rely on the broader public to inform policy decisions. Generative AI can flood these channels with convincing, personalized narratives.
- Social media: Generative AI enables bots to pose as humans and promulgate specified narratives as it interacts with users. For example, Chinese authorities have been known to hire internet commentators termed the "50 Cent Party" to spread official propaganda. AI enables such operations to be deployed at scale while removing language barriers.
- Academic publications: Abstracts written by ChatGPT have fooled scientists, raising concerns that sophisticated-sounding publications could be created at scale to advance political agendas, especially as scientific preprints have been cited in public discourse to substantiate policy ideas.
Some of these channels will be harder to filter. For instance, public feedback benefits from anonymity, so detection cannot rely on personal identification. Tweets and chatbots also contain short lengths of text that can diminish the reliability of AI detection tools, particularly as generative AI becomes more advanced.
(2) Taxonomy of AI-generated text detection systems
We review the types of AI text detection systems below, listing out their pros and cons.
The GPTZero team proposes combining approaches. For example, integrating AI-driven detection with output logs will lead to more comprehensive verification systems. Additionally, a system combining self-reporting with output logs can enable users to verify that a piece of content was typed and inputted by themselves. This verification proof can then be shared with others. In page 5, we discuss current efforts to build such a self-verification system at GPTZero.
(3) What are the costs of AI-generated content detection systems?
Mandating the widespread use of AI-generated content detection systems can be costly. First, certain types of AI detection, such as output logs, can be computationally and energy-intensive. Second, creating broad mandates for labeling content as AI-generated can cause warning fatigue as users become desensitized to labels. These systems can also lead to social backlash when it flags politicized content (similar to what is playing out with “sensitive content” warnings).
We recognize that detection errors can also be harmful. Type I errors (i.e., cases where human-written text is marked as AI-generated) can wrongly accuse individuals and companies. These issues are already happening in the classroom with AI checkers that were hastily released like Turnitin. Even worse, these detection systems have been found to be biased, disproportionately flagging non-native English speakers’ text as AI-generated.
Type II errors (i.e., cases where AI content is not detected) undermine the purpose of the detection tool and can be especially problematic if the errors are distributed unevenly. For instance, actors may find novel ways to fool detection systems that have previously been established to be highly reliable. In cases where AI-generated text causes harm, detection systems may wrongly attribute text to particular services–an issue that will need to be dealt with if the detection results are ever used as court evidence.
(4) How should the White House think about policy and technical interventions?
To successfully distribute AI-generated content, a malicious actor must 1) access AI tools for generating the desired content, 2) create or exploit an account on a distribution platform, 3) post the content successfully, and 4) have other users view it. Although no part of this process can be made foolproof, interventions can provide layered defenses such that lapses in one stage can be caught in others.
Figure 1: Our recommendations for PCAST by intervention stage
Stage 1: Content Creation. Policymakers should work with developers to make it more difficult for malicious actors to access LLMs that can generate disinformation. For instance, PCAST can recommend mandated safeguards that prevent the generation of disinformation, the use of chatbots in political contexts, or the production of hate speech. It can also propose limiting access to advanced (and less secure) systems by requiring strict user verification and output logging. While these restrictions are complicated by open-source models and LLMs may still be susceptible to “jailbreaks,” the most advanced and easily accessible tools are currently provided by commercial entities. These rules can make it more difficult for bad actors to deploy advanced language models at scale.
Stage 2: Identity Verification. Policymakers can change the incentives surrounding account creation and identity verification. As AI-driven content can rapidly scale, policymakers should require platforms to take greater responsibility for the number of malicious accounts. Currently, platforms are only indirectly incentivized by concerns about deteriorating platform experience to remove fake accounts. This may lose out against more direct financial incentives to inflate the platform’s number of users. A mandate requiring companies to explicitly decrease the number of bots, similar to what the FCC implements with telecommunication providers, could better align incentives with desirable social outcomes.
Suppressing fake accounts could be accomplished via identity verification, with strictness varying depending on the perceived cost of disinformation on the platform. For example, Twitter could further impede spam account creation by raising the bar for verification, mandating both an email and a phone number. Even more stringent measures, such as photos of real-world identification, may be appropriate for channels like academic publications.
Stage 3: Content Sharing. Platforms should employ verification systems to determine whether content was entered or typed by a human at the time of posting. From a technical angle, this could be implemented in three ways. (i) At a basic level, platforms could offer a simple check box to disclose whether content is automated. (ii) At a more sophisticated level, the platform could use CAPTCHA puzzles to raise costs, though human users might still get around this by copy-and-pasting from a generative tool. (iii) With still greater sophistication, the platform could analyze typing patterns or use camera monitoring to verify content as human-typed. The GPTZero team is building such a tool. It allows users to verify their online writing (Microsoft Word, Google Docs, social media posts, blogs, emails) as human-generated and create a shareable verification link for editors, audience members, etc. The GPTZero team has released its first version of this system as part of its Chrome extension, Origin.
Figure 2: GPTZero’s new writing report feature which verifies if text is human written
Stage 4: Content Viewing. Drawing on cybersecurity research, policymakers can require platforms to flag AI-generated content at viewing. The PCAST Working Group can suggest that platforms offer clear indications on whether a piece of content is AI- or human-generated, combining the AI detection systems described in section 2 with account and post metadata to increase confidence. When these verification systems agree on a classification, platforms can assign the corresponding label to the posts and consider algorithmically deprioritizing the content. De-priorization can slow the spread of disinformation, empirically spread through networks quicker than true information. Platforms can also make special algorithmic modifications for political information or advertising to increase the likelihood of real human interactions.
Again, none of these methods are foolproof, but they help raise the cost for malicious actors and work effectively in combination with other interventions. Furthermore, we note that not all accounts need to be human-driven. AI-generated content can be valuable. For instance, popular Twitter utilities like @threadreaderapp and @savemyvideo disclose that they are bots, so offering clear labels can enable these systems to continue operating.
Remediation. AI content detection systems may make mistakes. It is important for platforms to follow the AI Bill of Rights and the Santa Clara principles for content moderation, which emphasize the need for comprehensive disclosures (the amount and types of errors, the number of posts with each label, etc.) and responsive error-correction appeals processes. Disagreement between labeling systems suggests a greater likelihood of error. In these cases, the platform can label the posts as “in dispute” and initiate an appeals process (similar to what currently exists for content moderation). Unlike content moderation, however, where processes resort to human judgment, AI-generated content appeals processes will likely involve using additional AI-detection methods, such as ones too computationally expensive to apply to every post. The appeals process could also give content creators an explanation for why their post was labeled as AI-generated (e.g., the portions of text flagged by the detector) and the ability to rectify the results by offering an option to prove their content was human generated.
Authors:
Edward Tian, GPTZero
Justin Curl, Princeton Center for Information Technology
Sihao Huang, University of Oxford