Maintaining a Human-Centric Application and Selection Process in the Age of Generative AI
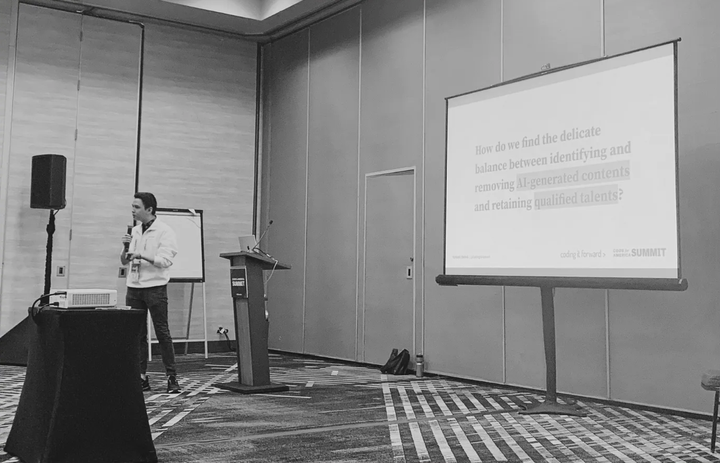
Editors Note: This post is a guest contribution from Yuyang Zhong, the program manager for Coding it Forward, first published here as a framework for using AI-generated content detection in their selective application process.
With the rise of generative AI (GenAI) tools like ChatGPT, candidates applying for jobs, internships, and fellowships are increasingly turning towards AI-generated content to speed up their application process. Since the introduction of ChatGPT in late 2022, Coding it Forward has seen a disproportionate amount of AI-generated content in application responses for our Fellowship, as documented in our 2023 Application Cycle Round-Up.
While Coding it Forward recognizes the power of AI to help us become better communicators, our application review process strongly emphasizes being human-centered — both in the application submission and the review process. With our application number more than doubling within two years, the rapid expansion of our applicant base has presented our team with a unique challenge and opportunity: how do we strike the delicate balance between identifying and removing AI-generated content while retaining qualified talent in our pool?
How do we strike the delicate balance between identifying and removing AI-generated content while retaining qualified talent in our pool?
What is Generative AI (GenAI)?
Generative AI is a technology that can generate coherent and (mostly) valid responses based on user inputs of just a few words. Its output could range from text, images, audio, and even videos. We first saw this with the rollout of DALL-E in 2021, an image generation engine that everyone at the time (myself included) wanted to get on the waitlist to play with. The pivotal moment came with the release of ChatGPT in 2022, where it gained massive popularity. Fast forward to just a year later, we see tools like ChatGPT, Copilot, and Gemini embedded into many fabrics of our daily lives, from writing content and codes to AI-powered chatbots for customer service.
What do we see in our application responses with the rise of GenAI?
Similar to what we’ve seen in our 2023 application cycle, our reviewers have identified a nonnegligible amount of AI-generated content in our 2024 applications. Even with a clearly defined policy on acceptable AI use, AI-generated content continued to be found. While we recognize the ease of using GenAI to write application responses, as novel and innovative as it may be, we lose the human touch in the process.
Coding it Forward’s Acceptable Artificial Intelligence Use Policy
With the emergence of generative artificial intelligence (AI) tools like ChatGPT, we underscore the importance of representing your own personal perspectives and writing. As you consider using AI tools to assist you in the preparation of this application, please note the following guidelines:
- Using AI to brainstorm, research, and copy-edit is acceptable. AI tools can help us become better writers, researchers, and communicators.
- Using AI to generate/compose partial or complete responses is NOT acceptable and may result in the rejection of your application. We do not believe that AI-generated writing genuinely represents who you are as a candidate.
When reading submissions myself, there are many occasions when I ponder: this piece of writing is cohesive and well-written, but it feels somewhat “grand,” and something’s amiss. In this instance, this gut feeling is often confirmed by the trends we see in this analysis, where we see detailed, tailored explanations with more straightforward diction that are more likely to be genuinely written by a human. AI is more likely to generate overly elaborative, hollow pieces of text with impeccable grammar and unimpeachable diction.
In partnership with GPTZero, we screened our application submissions to identify AI-generated content. We further analyzed the text contents to see the disparities in themes and word choices between human-written text and AI-generated text. Not surprisingly, we see very similar results to what we’ve found from our 2023 cycle.
In our application submissions, we asked our applicants to discuss their motivations for joining the Fellowship. Genuine responses typically touch on an applicant’s life experiences (school, college, family, student, career) and action verbs relating to service and learning (give, help, provide, thank, understand, learn). AI-generated responses, on the other hand, use big words without deeper elaboration. We see many adjectives to describe the Fellowship experience (profound, dedicating, excited, pressing, equitable, like-minded, educational, informed, firsthand, etc.), but many are without elaboration.
In our assessment submissions, applicants were tasked to walk through solving a technical problem similar to one a Fellow would work on during our Fellowship. We see the same disparity between genuine and AI-generated responses as we’ve seen in our application. Genuine responses focus on the scenario where applicants list requirements, state assumptions, and identify potential actions. AI-generated responses describe the issue, using grand and high-level words with little to no value in solving the actual problem.
Should you detect AI-generated content in your process? Here’s why we decided to do it.
As mentioned, AI is a complex field that disrupts our well-established approaches and practices. There isn’t a one-size-fits-all answer, especially given how quickly the AI landscape changes.
For us at Coding it Forward, we are building the infrastructure and resources to enable early-career technologists to innovate in government. We are looking for Fellows who are genuinely passionate about public service and technically competent; this sets us apart from a traditional internship program. We hold our applicants accountable for truthfully representing themselves, their passion, dedication, and expertise — which we believe cannot be well-demonstrated through AI-generated content.
Moreover, with limited resources, reviewers, and a growing applicant pool, it would not be fair, both to our reviewers and our applicants, to review an overwhelmingly large amount of similar-worded responses generated by AI as other genuine responses. We want to prioritize our attention and resources to applicants genuinely invested in our mission, passionate about our work, and may have spent hours crafting the best snapshot of themselves in an application, not a copy-pasted response generated by a chatbot in less than a minute.
Ultimately, for your organization, here are a couple of things to consider whether you need AI detection in your process:
- Resources and scale: How big is your applicant pool? How many staff members do you have available to review? How quickly do you need to turn around your reviews?
- Process flow: How extensive is your screening process? Are you just reading resumes, or do you have other components (essays, portfolios) to review? What content are you detecting?
- Objective: What are you looking for in a candidate? Is it relatively standard (technical abilities/skills) or personal (commitment, passion, interest)? Can what you are screening for be well-represented in an AI-generated response?
- Privacy and ethics: How do you plan to utilize the outcome of the AI screening? How should you preemptively discourage applicants from using AI if it is unwanted? Are there privacy concerns about utilizing a third-party service? How do you disclose the potential use of AI detection tools?
What are the implications of using AI detection in application and review processes?
As you consider implementing AI detection in your process, here are some guiding questions on the implications of using a detection tool.
- Determining the threshold: Many AI detection tools would output a confidence score between 0 and 100 on whether they think AI generated a piece of text. Some even go as far as scoring each sentence and paragraph separately. What is the cutoff level where you believe a piece of text is “too much AI?” Do you need to use the same threshold for different groups/pools of submissions, and why?
- False positives: No algorithm is perfect. What information do you have on the tool for flagging false positives, meaning that genuine writing is being flagged as AI? Are you comfortable with the tool’s statistics or suggested approach to mitigating false positives?
- Non-native English writers: There have been reports of AI detection models being biased against Non-native English writers. Is this something you can address from the analysis/outcome of the tool? Are there other considerations where non-Native writing may be more or less of a concern?
Again, it comes down to what pieces of text you are trying to run detection on and your goal for detecting AI and reviewing applications in the first place. At Coding it Forward, we always ask ourselves: “Can AI write a piece of text that truthfully responds to what we are asking?” If the answer is yes (often for questions related to technical steps and procedures), there’s little use in implementing AI detection. If the answer is no, AI detection is helpful, especially when answering questions about personal aspirations and background.
At Coding it Forward, we always ask ourselves: “Can AI write a piece of text that truthfully responds to what we are asking?”
That said, it is also important to ask the right questions in the first place. Are you more interested in learning how technically competent an applicant is or how the applicant can apply their technical knowledge to solve a specific problem? Both are about an applicant’s technical expertise, but one is about the “What,” and the other about the “How.”
What tools are available to detect AI-generated content?
Since the debut of ChatGPT in late 2022, many AI detection tools have become available. We mentioned in our previous blog that HuggingFace has a publicly available transformer model for text generated with GPT-2, which is rather outdated compared to GPT-3.5/GPT-4 nowadays. OpenAI (maker of DALL-E and ChatGPT) also failed to release its own AI detector, which did not prove to be accurate more than 25% of the time.
We’ve partnered with GPTZero since its founding in 2023 by Edward Tian. You can also check out a couple more tools and models listed on the Stanford study. In the near future, we also should see more ways to identify AI-generated content added directly into GenAI tools accurately, which reduces the need for a third party to develop AI detection tools without knowledge of the source algorithm. One example would be watermarking, where the AI models automatically embed specific word structures or image metadata to “stamp” an AI-generated watermark.
AI tools are rapidly changing, and as technologists, we must continue adapting as new technologies enter our larger ecosystem. This guide builds upon my lighting talk at the 2024 Code for America Summit of the same title. I hope this has helped you contextualize GenAI and ways we can prioritize genuine, human submissions over AI-generated content in application processes.