How Should Educators Approach AI and Academic Integrity?
A “Swiss cheese” strategy for AI in education means combining layered, imperfect tools to protect learning and integrity.
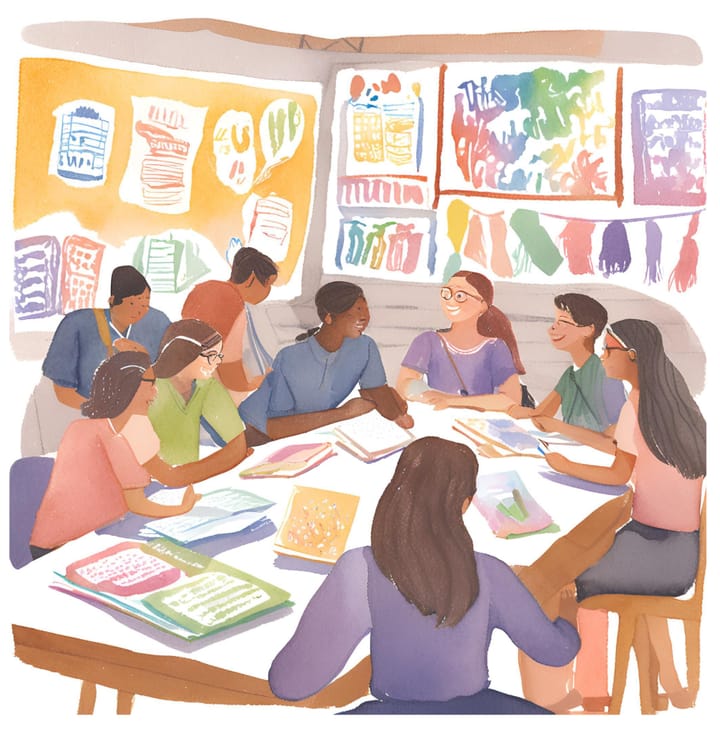
Anna Mills started out as a skeptic – or, in her own words, “argued against use of AI detection in college classrooms for two years.” Now, her perspective has shifted. As an English instructor at the College of Marin and an expert in AI pedagogy, she’s asking the question many educators are wrestling within: how can we integrate AI in ways that support learning, without compromising academic integrity?
Her answer is to take inspiration from Phillip Dawson and use a multi-layered, practical approach – like “Swiss cheese” – a term often used in safety science.
What is the Swiss Cheese approach?
Imagine layering slices of Swiss cheese to create a protective wall, with each slice symbolizing a safety measure or rule. Every slice has holes (weak spots) where errors might sneak through. When the holes in several slices line up, they form a direct path, allowing an accident to occur. This idea captures the essence of the Swiss cheese model, developed by James Reason to show how errors happen in complex systems with multiple points of failure.
Applied to AI in education, the model suggests that no single strategy (not even AI detection tools!) will be airtight. But by combining multiple imperfect methods, educators can reduce the chances of academic dishonesty or learning loss slipping through the cracks. One slice might be AI detectors (used cautiously), another might be intentional assignment design, another might be a conversation about ethics, and another might be reflection prompts.
None of these are perfect on their own. But together, they form a more resilient system.
AI and the Risk of Learning Loss
“Most teachers don’t want to put their energy into ‘policing’ students,” Mills acknowledges. “It’s not why we went into teaching.” But she’s also wary of the cost of ignoring AI misuse. “We need ways to reduce potential learning loss and unfairness.”
The risks go far beyond cheating – when students use AI in ways that shortcut learning, there’s a breakdown in the learning process itself. As one teacher using GPTZero put it:
“I’m worried that students will lose the ability to express original ideas in their own voices, and think that they are just a conduit for taking in information and regurgitating it.”
In other words, if students outsource too much of their thinking, writing, and problem-solving, the essential foundation of education begins to erode.
Recent examples show just how widespread the issue might be. “On March 26, 2024, philosophy professor Jonathan Birch posted on X, reporting secondhand on a class where 92% of students admitted to using ChatGPT when it wasn’t permitted,” she says. “That may not be accurate, but even if the percentage is a tenth of that, we have a problem.”
The issue, she argues, isn’t just about academic dishonesty but about the loss of essential skills and the credibility of our educational systems.
Dr. Tricia Bertram-Gallant puts it plainly: ignoring the impact of GenAI tools on your course learning outcomes undermines everything. “You’re not teaching the skills/knowledge you think you are; students aren’t learning what we intended; we aren’t evaluating what we think we are; students don’t have the knowledge & skills we say they do.” The implications affect everything from teaching practice to degree value.
Building Your Own Swiss Cheese Model
Instead of relying on a single solution, Mills advocates for a toolkit of responses – each a layer in your Swiss cheese model.
She shares that she’s a member of the MLA/CCCC Joint Task Force on Writing and AI, a group that has put out strong cautions about it in their working paper on Generative AI and Policy Development. Their stance is clear: "Tools for detection and authorship verification in GAI use should be used with caution and discernment or not at all."
They go on to ask: "For those who decide to use AI detectors, please consider the following questions: What steps have you taken to substantiate a positive detection? What other kinds of engagement with the student’s writing affirms your decision to assign a failing grade outside the AI detector’s claim that the text was AI generated?"
They also stress that “any technological approaches to academic integrity should respect legal, privacy, nondiscrimination, and data rights of students.”
Other ‘slices’ in the Swiss cheese model might include the below.
Designing assignments harder to outsource to AI
One of the most effective ways to discourage over-reliance on generative AI tools is to design assignments that are deeply personal, process-based, or tied closely to specific classroom contexts. For example, instead of assigning a generic essay question that can easily be answered by AI, ask students to reflect on a class discussion, incorporate feedback from a peer review session, or connect course concepts to their own lived experience.
You could also require multiple drafts with checkpoints along the way, including rough outlines, annotated bibliographies, and reflections on feedback received. These types of assignments don’t just make it harder to copy-paste from a chatbot – they also reinforce authentic learning by helping students engage more deeply with the material and their own thinking.
Creating space for honest conversations
Instead of relying on assumptions – and assuming students will either cheat or stay within the lines – consider opening up a dialogue about how AI is actually being used in their writing process. Normalize conversations around questions like: What kind of tools are you using to brainstorm or revise?
When you model transparency and curiosity (rather than suspicion), students are more likely to engage – and be open themselves. Giving them clear expectations about when AI tools are allowed (and when they’re not) is the key. So is creating a space where students feel comfortable asking questions, making mistakes, and learning how to use these tools responsibly.
Encouraging metacognition
Ask students to explain their choices and describe their writing process – with simple measures like reflective cover letters, process notes, or revision memos which can prompt students to think more critically about how they approached an assignment. What decisions did they make as they wrote? Where did they get stuck? What feedback shaped their final draft?
These metacognitive tasks not only give you an insight into how a student is learning but also help students become more aware of their own learning habits and processes. While this approach can take more time, it supports an important culture shift: one in which students feel both accountable and supported in their learning.
Looking Beyond AI Detectors
Despite their appeal, AI detection tools are not a silver bullet. They can provide signals but not certainty – and their use risks false positives, not to mention damaged trust as there becomes a move towards surveillance rather than focusing on learning.
Instead of relying on detection, Mills encourages educators to focus on designing learning experiences based on curiosity, connection, and critical thinking. Detection might feel like a shortcut, but it’s ultimately just one slice of the cheese - full of holes if used alone.
The more intentional layers we build in – from pedagogy to policy to honest dialogue – the better we can protect the integrity of learning in the classroom.